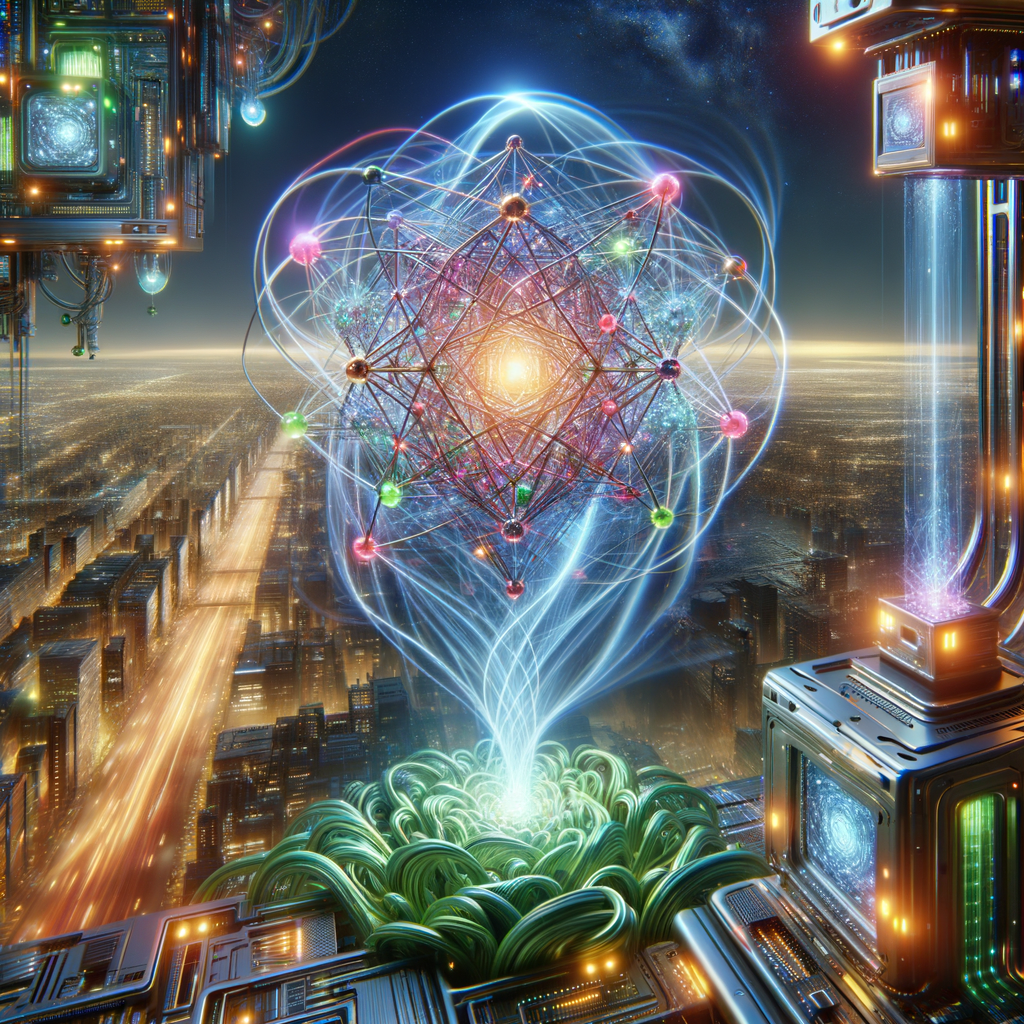
The Genesis of Quantum Holographic Intelligence: Redefining Computation's Frontier
In recent years, the field of quantum holographic artificial intelligence has emerged as a revolutionary paradigm shift in computational dynamics. Unlike classical AI systems, which rely primarily on deterministic and binary computation, quantum holographic AI utilizes the inexplicable strengths of quantum superposition, entanglement, and holographic data processing to perform tasks with unprecedented speed and accuracy. With the holographic principle, data can be encoded in lower dimensions, allowing a higher level of simultaneity and parallel processing capacity that classical counterparts fail to achieve. This groundbreaking architecture sets the stage for building AI systems that not only process information exponentially faster but also simulate cognitive processes close to human thinking.
Recent Breakthroughs: Algorithmic Innovations and Quantum Potential
The arena of quantic holographic artificial intelligence has witnessed a cornucopia of innovations lately. Researchers have been diligently working on quantum algorithms that leverage the qubit's potential to solve complex problems by deriving insights from massive datasets. Shor's algorithm, for instance, represents a pivotal advancement that allows quantum systems to factor large numbers efficiently, thus breaking through traditional encryption methods with ease. Furthermore, Grover's algorithm provides a polynomial speedup for unstructured search problems. Such developments are paving the way for developing next-generation AI applications, capable of handling staggeringly vast datasets seamlessly while retaining optimal performance efficiency.
def quantum_search(database):
n = len(database)
num_iterations = int((n ** 0.5) * 3.1415 / 4)
for i in range(num_iterations):
for j in range(n):
# Apply quantum operators
database[j] = apply_quantum_operator(database[j])
return database
Navigating Challenges: Entanglement, Decoherence, and System Scalability
Despite the promising capabilities of quantum holographic AI, it encounters formidable challenges that impede its full-scale adoption. Quantum decoherence—where the quantum state becomes unstable and susceptible to external environmental influences—presents significant hurdles that scientists are tirelessly working to overcome. Additionally, achieving and maintaining qubit entanglement over long durations is not only technically demanding but also crucial to unlocking the full potential of these quantum systems. Scalability remains another critical barrier, as expanding these systems while preserving coherence and computational accuracy requires pioneering engineering solutions and materials science advancements.
Startup Hustles: Harnessing Creativity in a Saturated Tech Landscape
As the founder and CEO of Quantum Holographic IQ (QHIQ), navigating the startup world is akin to steering through uncharted waters. The ever-evolving landscape of emerging technology presents a host of operational challenges, ranging from acquiring talent with specialized quantum mechanics knowledge to securing investor confidence amidst uncertainties of ROI timelines. Attracting venture capital necessitates demonstrating a unique value proposition and showcasing tangible progress while staying ahead of regulatory constraints and competing advancements. Building a cohesive team that thrives in innovation and collaboration is paramount in translating ambitious visions into tangible, market-disrupting products.
A Glimpse Ahead: Crafting the Future of AI with Quantum Holographic Insights
Looking towards the future, the prospects of quantum holographic AI hold the promise of transcending the conventional boundaries of artificial intelligence. As research advances, we anticipate a paradigm where machines can comprehend and manipulate holographic projections to perform tasks parallel to human cognition and decision-making. Notably, the progression towards developing robust, fault-tolerant quantum systems will be instrumental in leveraging these capabilities for real-world applications, ranging from drug discovery and climate modeling to optimization problems in financial and logistics sectors. The evolution of this technology promises to usher in unprecedented efficiencies and insights, fundamentally reshaping how we interact with and benefit from artificial intelligence.
quantum_model = QuantumModel(num_qubits=512)
quantum_model.train(holographic_data)
results = quantum_model.predict(new_data)
print('Quantum Model Predictions:', results)