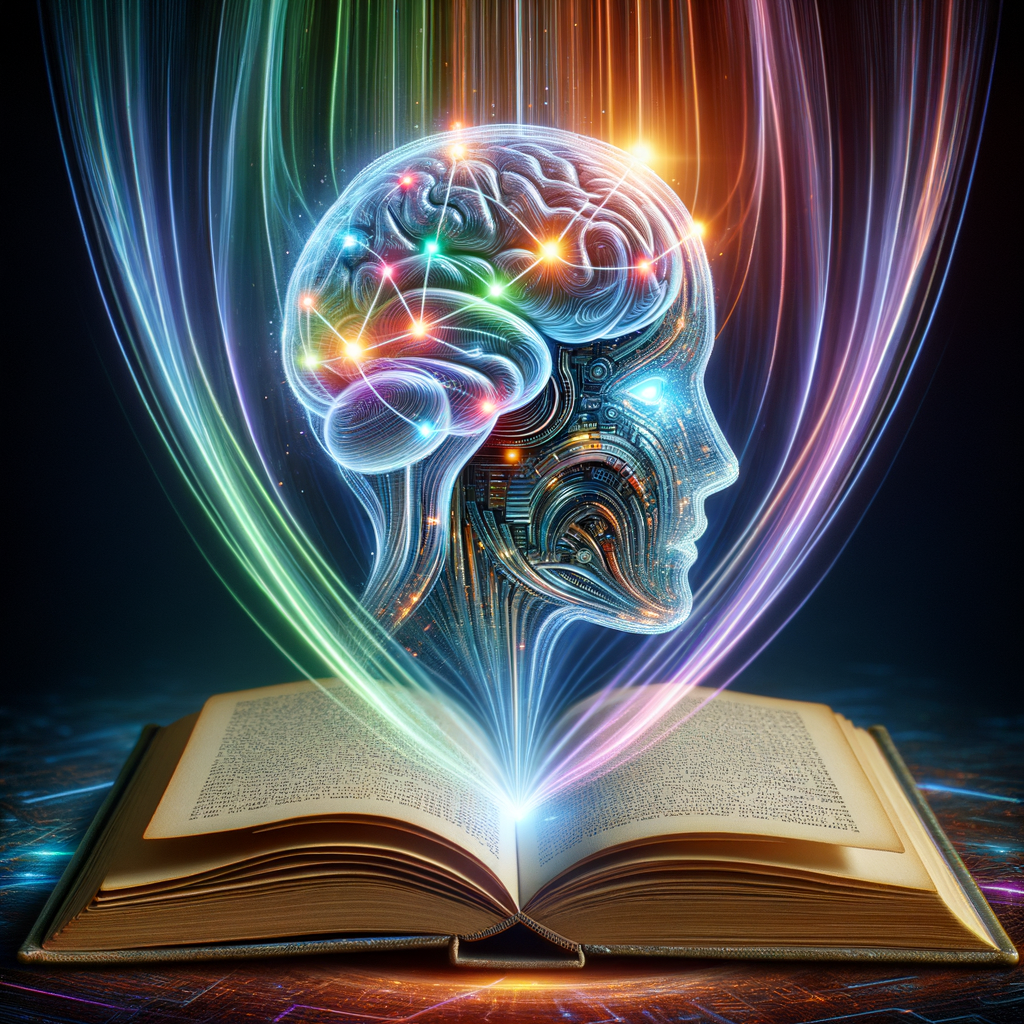
Introduction
The technology landscape has been evolving at a rapid pace, and one area that arguably stands out the most is Quantic Holographic Artificial Intelligence (QHAI). With revolutionary potential to redefine the concept of computing and intelligence, QHAI marries the science of quantum computing and holography with the tenets of AI, potentially bringing about drastic changes to data processing, machine learning, and more.
Defining QHAI
To understand QHAI, we need to grasp its two primary features - Quantum Computing and Holography. Quantum Computing harnesses the principles of quantum mechanics to process data exponentially faster than classical computers. Holography, on the other hand, uses interference and diffraction to record and reproduce a 3D light field, yielding 3D representations. QHAI leverages these enabling technologies, aiming to create AI models capable of exponentially high processing capabilities and 3D spatial recognition.
Recent Advancements
The past decade has seen substantial progress in QHAI. From Google's Sycamore processor, achieving 'quantum supremacy', to our own state-of-the-art, holographically enhanced quantum neural networks at Quantum Holographic IQ, we are truly living in exciting times.
Challenges and Future Perspectives
However, QHAI is not without its challenges. Technical obstacles such as quantum decoherence, maintaining holographic data coherence, and the lack of standardization frameworks are key issues. Looking forward, we are working on methods and techniques that can stabilize and control quantum states as well as create more effective holographic techniques. The goal? To bring about the maturation and mainstream adoption of QHAI.
Technical Insight and Code Samples
To give a glimpse into what we're working with, let's consider how we might create a simple quantum circuit.
from qiskit import QuantumCircuit, transpile, assemble, Aer, execute
from qiskit.visualization import plot_bloch_multivector, plot_histogram
# Create a Quantum Circuit acting on a quantum register of three qubits
circ = QuantumCircuit(3)
# Add a H gate on qubit 0, putting this qubit in superposition.
circ.h(0)
# Add a CX (CNOT) gate on control qubit 0 and target qubit 1, putting the qubits in a Bell state.
circ.cx(0, 1)
# Run the quantum circuit on a statevector simulator backend
simulator = Aer.get_backend('statevector_simulator')
result = execute(circ, simulator).result()
statevec = result.get_statevector()
# Returns a dict of the counts
counts = result.get_counts(circ)
# plot histogram
plot_histogram(counts)